FinanceGPT Labs Whitepaper
Large Quantitative Models: A Generative AI Approach to Overcome Predictive AI and Large Language Model Challenges in Stock Price Prediction and Financial Forecasting
ABSTRACT
This whitepaper presents FinanceGPT, a novel Variational AutoEncoder Generative Adversarial Network (VAE-GAN) framework designed to address the limitations of Large Language Models (LLMs) and traditional predictive AI in financial forecasting and stock price prediction. The paper introduces Large Quantitative Models (LQMs), a new class of pre-trained generative AI models, tailored for quantitative finance applications. LQMs capture the intricacies of quantitative relationships and extract insights from complex financial data, addressing the challenges of data volatility, limited historical data, non-linear relationships, and overfitting. The paper explores the architecture, training, application, and advantages of LQMs, and their potential to improve AI-powered financial analysis and decision-making. It also discusses the role of FinanceGPT within the broader context of Artificial General Intelligence (AGI), highlighting its potential contributions to the development of AGI in finance and investments.
Author: Phiwa Nkambule
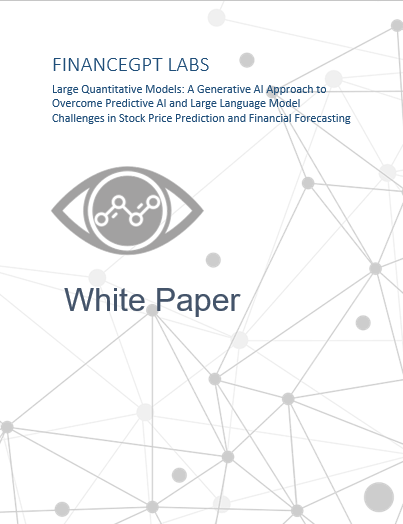